ABU DHABI (ALETIHAD)
Researchers from the Mohamed bin Zayed University for Artificial Intelligence (MBZUAI) are using a novel combination of federated and reinforcement learning to make smart grids more secure, efficient, and reliable.
If governments and corporations are to meet their commitments to achieve Net Zero Emissions by 2050, they will need to help drive a wholesale energy transition that will lead to the widespread adoption of renewable sources of energy.
So far, attention has focused on electrification, the construction of wind and solar farms, and the adoption of electric vehicles, but in isolation these efforts will prove insufficient in the fight against climate change unless authorities fail to tackle the infrastructure issue that lies at the heart of the forthcoming energy transition.
If the transition to renewable energy is to succeed, the world needs a new generation of transmission and distribution infrastructure for electricity that can cope with the specific demands of renewable energy-based electrification. Cue the need for smarter grids.
Designed long before renewable energy became a transformative reality, the existing energy grids deliver power in one direction – from the point of its generation across transmission networks and throughout distribution networks – to industrial and domestic consumers while maintaining a careful balance between energy supply and demand.
Outdated Infrastructure
But with widespread electrification, the accelerating deployment of renewable energy, and the increasing emergence of localised power generation through domestic solar panels and wind turbines, the world’s legacy grids are becoming increasingly outdated.
Smart grids are flexible enough to match the increasing demand for electricity with sudden and often unexpected fluctuations in energy demand and supply, a flexibility that requires the ability to monitor, measure, and assess the flow and availability of energy across distribution grids in real-time to prevent wider system failures and power outages.
While this may sound like an esoteric concern, the challenge is actually central to the success of the energy transition. In 2020, a team from Princeton University predicted that the US would need to invest $360 billion to make its antiquated energy transmission system fit for the 21st century.
To effectively balance the highly variable energy flows that are the product of multiple renewable energy sources and accommodate fluctuating consumption levels, highly sophisticated computing power must be deployed at the edge of existing grids – smart grids – that allow renewable energy to behave with the same predictability as traditional power.
Luckily, help is at hand thanks to research that is being carried out at MBZUAI, the world’s first academic institution dedicated to AI.
Safeguarding user data
“Every AI has to be trained on a lot of data to make it reliable. But we cannot train an AI in a classical way to understand energy consumption because it would require gathering data that people might not want to share because it breaches their sense of privacy,” explained Professor Martin Takáč, Deputy Department Chair of Machine Learning, and Associate Professor of Machine Learning at MBZUAI. “That requires us to train the AI in a smarter way.”
Takáč’s team are applying a novel technique that combines reinforcement and federated learning to efficiently optimise smart grids, while enabling new applications and safeguarding the anonymity of user data.
Reinforcement learning is a type of machine learning that trains algorithms to learn from their environments, while federated learning adopts a decentralised approach that does not require direct access to data. Instead, it uses raw data gathered from more than one place and processes it at its source, whether that is on a phone, laptop, thermostat, or even a satellite. In doing so, MBZUAI are able to access a new stream of data that is currently underutilised.
“Imagine you have a house with solar panels and batteries, and you want to know when to use your energy or to sell it [back to the grid],” Takáč said. “The energy price will depend on the demand, so whether you are an individual or a company you need AI to help you understand what’s happening with the price to be able to make an optimal decision.”
The professor explained that using AI to solve such a problem is difficult because when it comes to assessing the potential variables involved in such a calculation “everything matters” and there is also a high degree of unpredictability, something computer scientists refer to as stochasticity.
Federated learning has been successfully deployed in the healthcare sector, where it has enabled companies to train decentralised AI models without the need for sharing medical records or breaching patient confidentiality. Takáč’s research shows it also has the potential to assist in the development of a new type of electricity distribution infrastructure that is capable, not just of harnessing the benefits associated with renewable power, but of encouraging consumers to make more sustainable choices.
Encouraging sustainable choices
“If we have the data that enables us to understand how people are really using electricity, then maybe we can also use AI to help change people’s consumption patterns,” explained Nicolas Mauricio Cuadrado Ávila, a graduate student in Takáč’s team, who first encountered these issues in his home country of Colombia. “Small changes in energy pricing between day and night could be used to determine when people decide to do their laundry, for example, which is actually a big deal because such changes in behaviour can be used to flatten demand curves, which means you don’t need to build so much energy generating infrastructure.”
The use of variable time-of-use (TOU) electricity rates to encourage electricity use at times when there is less demand is well understood, as is its positive impact on grid loads and utility bills, but they are also important for encouraging daytime energy use when the cost of solar power is at its lowest because solar energy generation is at its peak.
The other reason for encouraging energy consumption patterns that mirror the availability of renewable energy is to enable energy supply companies to ensure that they have sufficient power to meet predicted energy needs – especially peak loads – as well as unanticipated spikes in demand.
“The system is very complex,” explained Takáč’s colleague and co-researcher, Dr. Samuel Horváth, Assistant Professor of Machine Learning at MBZUAI. “That means that none of the standard solutions we use in control theory work in this situation. And as a result of the highly stochastic nature of the way that people use energy, the extra challenge is to maintain the privacy of the energy user.”
The solution that Takáč’s team is developing is to make the whole process of data analysis private by distributing an AI-based model to individual users that then operates on private devices before being returned to the grid as an updated model. The data itself is never shared.
“We do all the learning on your device with your data,” Horvath explained. “That means we don’t look at an individual’s data, we look at aggregated updates from all of the models rather than the data itself.”
In September 2023, the International Energy Agency (IEA) published an update to its landmark 2021 report, “Net Zero Emissions by 2050: A Roadmap for the Global Energy Sector”, as part of its support for the Global Stocktake (GST). One of the most anticipated outcomes of the COP28 UAE, the GSTwill assess the collective progress that has been made in achieving the goals set out in the 2015 Paris Agreement.
“Ramping up renewables, improving energy efficiency, cutting methane emissions and increasing electrification with technologies available today deliver more than 80% of the emissions reductions needed by 2030,” the IEA concluded, stating that even though “the path to 1.5 degrees Celsius has narrowed”, the dramatic growth in clean energy was keeping it open.
Smart grids will play a fundamental role in enabling the further expansion and impact of renewable energy, not just by helping to manage the necessary energy transition and reducing the need for costly new grid infrastructure but by helping to make grids more resilient, reliable, and secure.
AI-empowered smart grids: accelerating the energy transition
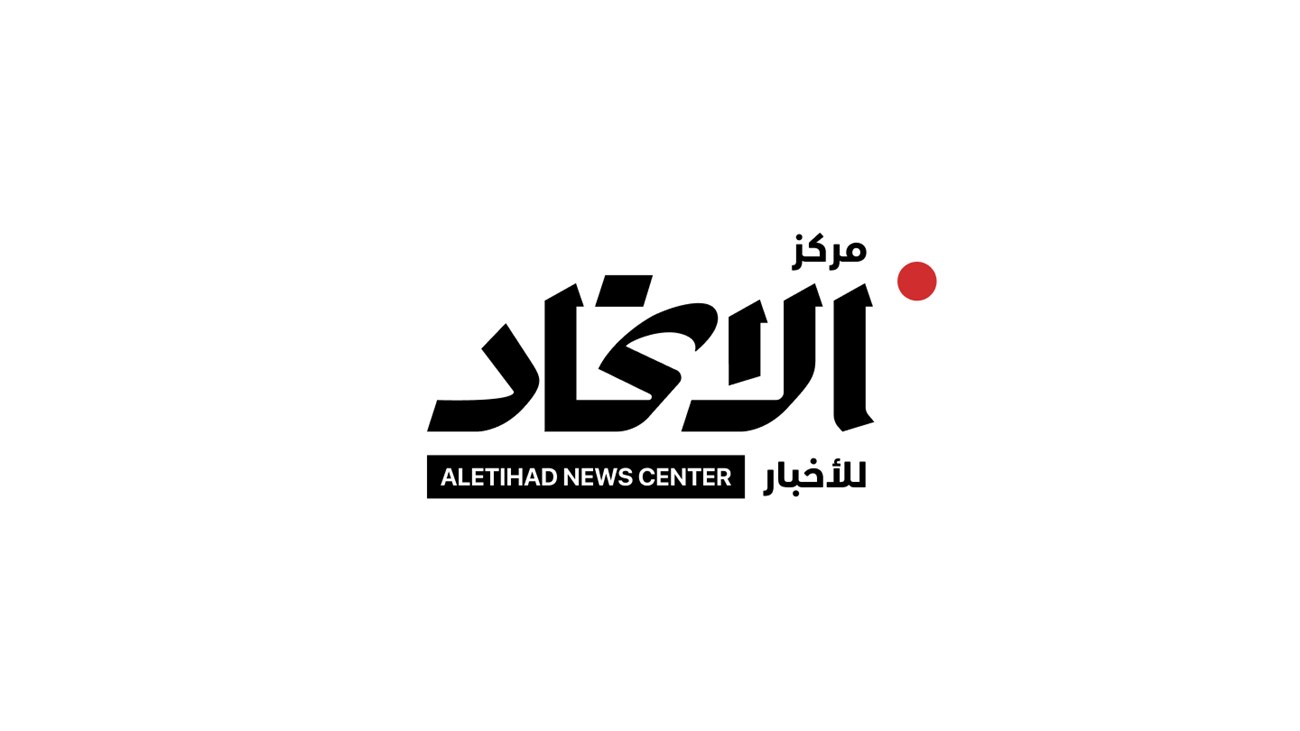

Source: Aletihad - Abu Dhabi